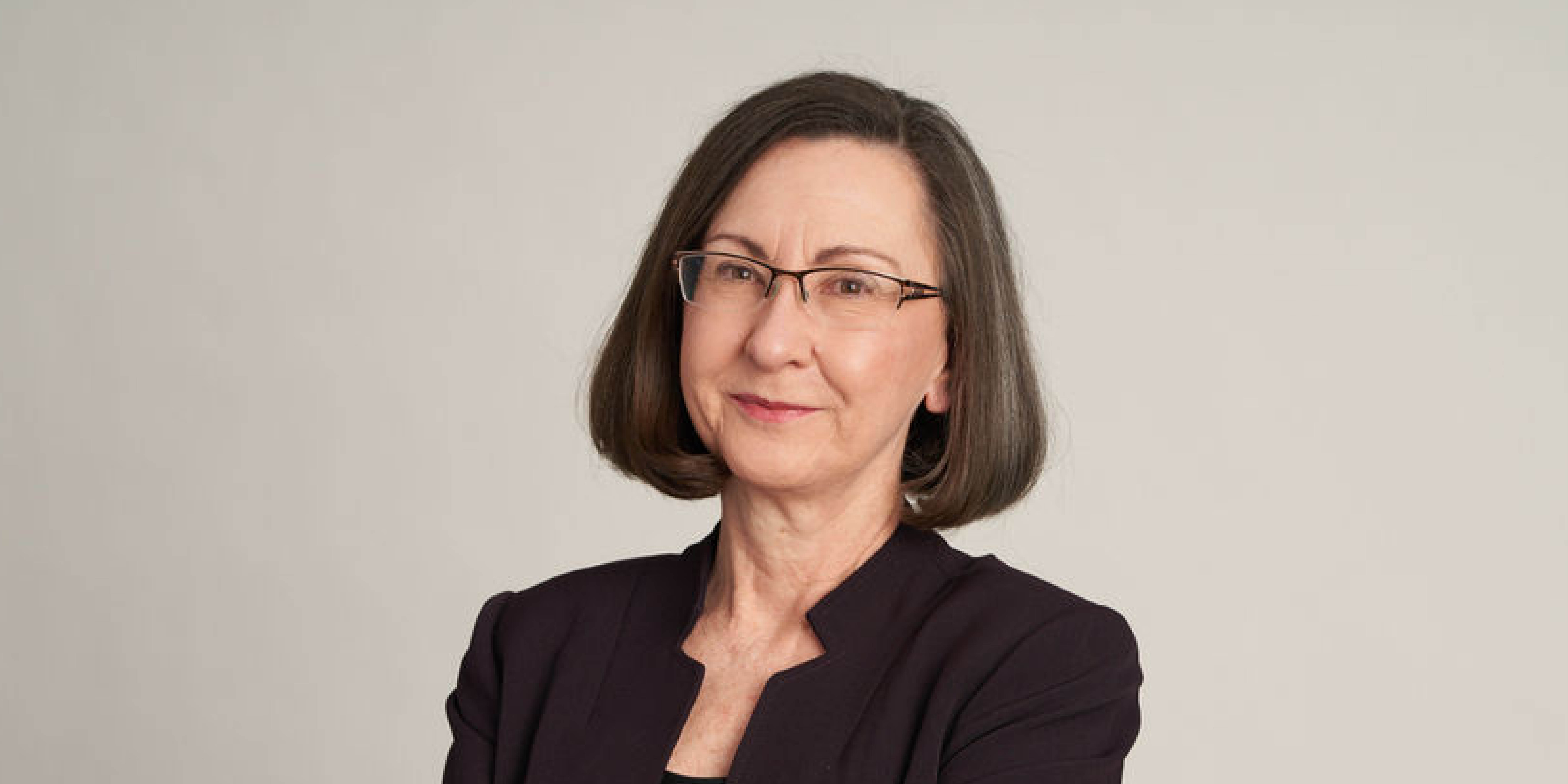
Federal government organizations should become early adopters of data mesh because most stand to benefit from pivoting from a traditional approach to data mesh as soon as possible.
By Cindy Walker, VP, Data Management & Analytics Center of Excellence
What is Data Mesh?
Data mesh is a new approach to collect, manage, and share data for analytical use cases at scale. Zhamak Dehghani of Thoughtworks began evangelizing data mesh in 2019 as a paradigm shift that was needed to overcome limitations and failure modes of traditional data management approaches. Data mesh is a not a tool or a product that you can purchase. It is an approach that has four basic elements that enable a robust, governed, data product marketplace where providers and consumers can interact directly:
- Distribute ownership of data to the business domains where the data comes from and who understand the business context and rules that should apply to the data in their domains.
- Make those teams accountable for product thinking and responsible for serving data from their domains as a high value product via a distributed data marketplace to consumers.
- Make it easy for those domain teams to be self-sufficient by providing a self-service infrastructure as a data platform that does not require data tool specialists to manage the data product life cycle or to discover and use data products.
- Use a new operating model of federated data governance to define and automate the enforcement of both global and local policies and rules within each individual data product.
My “Ah Ha” Moment
When I first read Dehghani’s original data mesh blog post, it immediately resonated with me. To be honest, it more than resonated. I felt like I had been struck with a lightning bolt, and I had a combination ah ha/duh moment:
- OF COURSE! It makes sense to embrace product thinking and domain data ownership to ensure trustworthy data for analytics is managed as a product to be served to data consumers – not just an asset to be collected!
- And YES! A data product is a new kind of architectural thing – it should be immutable, observable, discoverable, accessible, and governed deployed using distributed architecture and microservices models!
- And DUH! Why did we go all monolithic and centralized with our data architectures and data governance operating models and stay there, especially when the value and power of distributed architectures and microservices played out before our very eyes!? Why have we not used those same best practices applied to data before now??
Digging Deeper into Data Mesh with Federal Government Needs and Challenges in Mind
As a long-time data management professional with almost 40 years and dozens of Federal Government data projects under my belt, I wanted to dig deeper to explore the promise and potential pitfalls that data mesh posed for federal clients. So, after my initial ah-ha/duh moment, I immersed myself in everything I could find about data mesh. I also had the benefit of an investment of capital resources from my company, Octo, to focus a team on developing data-mesh innovations around the self-service infrastructure as a data platform and federated, computational governance elements of data mesh. Finally, I was delighted to have an opportunity to begin working with two of my federal clients to help them pivot to data mesh.
Three-Part Blog Series on Data Mesh for Federal Government Organizations
Now, about a year into these research, exploration, and early adoption efforts, I have come to some important – and I hope valuable for Federal Government organizations – conclusions that I will share in this three-part series of blog posts.
This first post is dedicated to the conclusion that many Federal Government organizations stand to benefit from being early data mesh adopters, even though data mesh is – according to Jhamak – “still fairly nascent in its evolution.” I’ll devote the second part in this series to common misconceptions about data mesh that can stymy Federal Government adopters. In the third post, I’ll address a low-risk way for Federal Government organizations to get started with data mesh and reap immediate rewards.
Part One: Federal Government Organizations Stand to Benefit from Being Early Data Mesh Adopters
Pivoting to Embrace Domain Data Ownership and Data Product Thinking Can Yield Significant Value Immediately with Minimal Downside Risk
The promise of data mesh for Federal government organizations is significant. Most stand to benefit from pivoting from their current approach to a data mesh. Today, most Federal Government organizations want to drive innovation and become data driven, and they are not afraid to make huge investments in technology to try and achieve aspirational data goals. Many have invested billions of dollars in machine learning/artificial intelligence (ML/AI) capabilities to support the use of data analytics for data-driven decision making, yet they are still struggling to source, manage, access, and protect trustworthy analytical data at scale. As a result, most are not realizing maximum value from their significant and increasing investments in data analytics, and their data value to investment ratio is low. This disappointing return on investment (ROI) is not due to an absence of high-level support within the organization. In fact, in most federal agencies, it is the Chief Data Officer (CDO) or leaders at the highest levels of the organization pushing for change needed to accelerate innovation and realize data advantage. In my opinion, there are two primary reasons that federal agencies are not maximizing data value to investment, and both can be remediated by pivoting to data mesh:
1) Over-emphasis on data as a strategic asset with a focus on collecting and harmonizing at the enterprise level rather than a push of value mentality with the emphasis on individual data products that delight data consumers requires high investment with low return in terms of mission impact. Agencies can amass huge volumes of data in a data lake and expend a great deal of resources to accomplish that with limited value in terms of data consumers being able to discover, understand, and use that data to address mission questions. At its core, data mesh facilitates a fundamental shift from considering data as a strategic asset to managing and sharing data as a usable and valuable product. It also establishes a distributed data architecture of data products that leverages microservices to make data products shareable, secure, visible, and trustworthy. This means that just flipping the emphasis from “asset to collect” to “product to serve” can immediately yield more value from investment. Embracing the logical architecture of the “new” data product architectural quantum helps guide the design and development of high-value data products.
2) Separation of data for analytics from the domains that create the data creates a schism between operational and analytical dimensions of data and means that the important work of serving customers becomes disconnected from the domain-knowledgeable resources. Making domains responsible for serving and accountable for delighting consumers begins the important paradigm shift to thinking about how data will be used rather than where it will be collected. Product thinking helps eliminate and prevent data silos and establishing a data product owner role helps ensure data products deliver high mission value.
Being an early adopter means that federal organizations can begin to embrace these two core data mesh principles and realize value sooner with minimal risk. There is low risk because adopting these principles requires an organizational shift and shift in distribution (not addition) of people resources. It does not require any major technology acquisitions or major application redesigns. Being an early adopter means federal agencies can eliminate the central data team middle-man, thereby removing a bottleneck; they can embed ML/analytics capabilities within each domain; and they can enable value-driven peer-to-peer consumption of data for analytical use cases. This strategy also ensures mission continuity in the face of continuous change by focusing on change locally within a domain.
In Part Two of this blog post series, I’ll address common misconceptions about data mesh and dive into two areas – Federated Computational Governance and Self-Service Infrastructure as a Data Platform -where the misunderstanding is rampant and can stymy federal government organization adoption of data mesh if not dispelled. In the meantime, you might be interested in Jhamak Dehghani’s latest feedback in this post from Monte Carlo data where she dispels some of the misunderstandings that have sprung up around data mesh.
For more information about Octo’s data mesh solution, reach out to cto@octo.us.