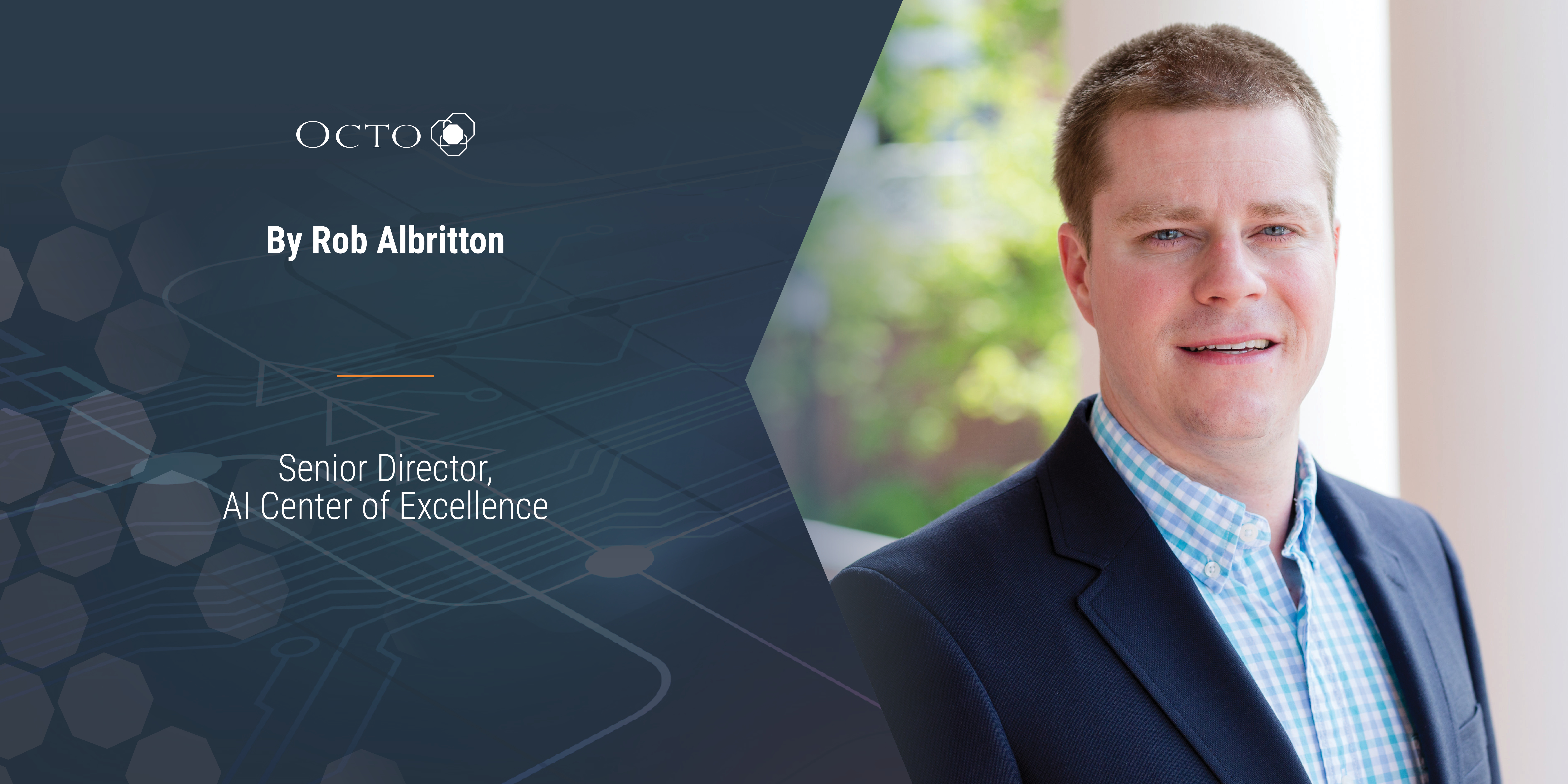
At this point in the evolution of AI, building mission-specific models is the most effective means of developing optimally effective AI solutions. There is no cookie-cutter solution.
By Rob Albritton, Senior Director, AI Center of Excellence
If you’ve been tracking AI, you will see many companies advocate using a single machine learning (ML) model or AI solution to address a variety of data repositories. But there’s a problem with the idea that a single model can address multiple datasets: AI doesn’t work that way. Because ML models require training on specific datasets and the incorporation of other criteria relevant to an enterprise’s mission to be truly effective, model generalization is exceedingly difficult. This is especially true with unique datasets and mission objectives in highly specialized enterprises like the Department of Defense (DoD), the Intelligence Community (IC), and the medical industry. A model trained to work on one dataset and addressing a particular mission outcome simply won’t be as effective when applied to another. It is for this reason Octo believes that, at this point in the evolution of AI, building mission-specific models is the most effective means of developing optimally effective AI solutions that support achieving mission objectives.
The difference is in the data
Training AI models gets even more specific when you try to reuse models built for agencies with vastly different missions and data formats. For example, analysts cannot easily take DoD or IC datasets and apply them to the Department of Health and Human Services (HHS) or other civilian agencies where the differences in datasets from DoD or IC data sets is extreme. There may be pieces of a particular model you can reuse, but likely very little of it will apply. For the most part, you need to understand the customer’s needs, data, and use cases to be able to build them the most effective AI model.
Customization is the cure
The best case scenario for creating a tailored, impactful AI solution designed to help achieve mission objectives can be found in the U.S. Army’s Integrated Visual Augmentation System (IVAS) program. Microsoft and the Army are resurrecting an old concept, Soldier-Centered Design, which is essentially human centered design specifically for Army. The Army has active duty soldiers sitting on-site at Microsoft’s offices in Redmond, Washington as they develop AI technology. This way, the developer gets feedback from someone who will actually use the technology in near-real time. The soldiers explain what they will use and what they won’t. Then they take it a step further as a team. Developers and engineers take their technology to the field and conduct realistic military exercises, soliciting feedback in operationally realistic settings for Army civilian engineers and Microsoft to learn what works, what doesn’t. They then use an iterative process to make changes that continually progress toward the Army’s goal of achieving overmatch over near-peer adversaries by providing soldiers with next generation AI-enabled situational awareness.
To be successful, developers need to observe end users in real life. You can’t sit in a lab and build technology for the military and expect it to be implemented. Technology built in a vacuum is destined to sit unused in a connex container. You have to see how warfighters, analysts, and other potential end-users do their jobs. You have to go to a theater of operation or exercise, understand how missions are planned and conducted, learn what happens in after action reviews, and then, as a tech expert, once you understand the domain, plug AI into gaps where you can see the technology would be beneficial.
Most companies still don’t employ such an integrated, user-centered approach enough. Processes tend to be stove-piped and insular. On the research and science side, there are labs with technologists who often conduct ample research, but they never leave their lab. The science and technology are strong, but they build a prototype first and then look for someone to use it rather than getting connected with end users from the start. Acquisitions personnel might actually buy the technology, but they don’t understand how it will be used in the field. So even if the prototype is adopted, it sits in a container and is never deployed.
Octo works to overcome these trends in AI development, trends that result in AI technology that doesn’t work the way it should (because the models were not trained on mission specific datasets) or is not used (because the end user was never part of the development process). Octo’s oLabs AI Center of Excellence is a rapid prototyping organization where mission domain experts, academics, engineers, thinkers, and doers come together to build and rapidly advance mission-specific and user-focused AI, not cookie cutter AI. By understanding the mission use case and differences in datasets and by working directly with warfighters and other end users, our expert teams ensure end users can meet mission objectives.
For more information on our AI solutions and approach, visit oLabs.